The Role of Generative AI in Creating Personalised Banking Experiences
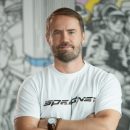
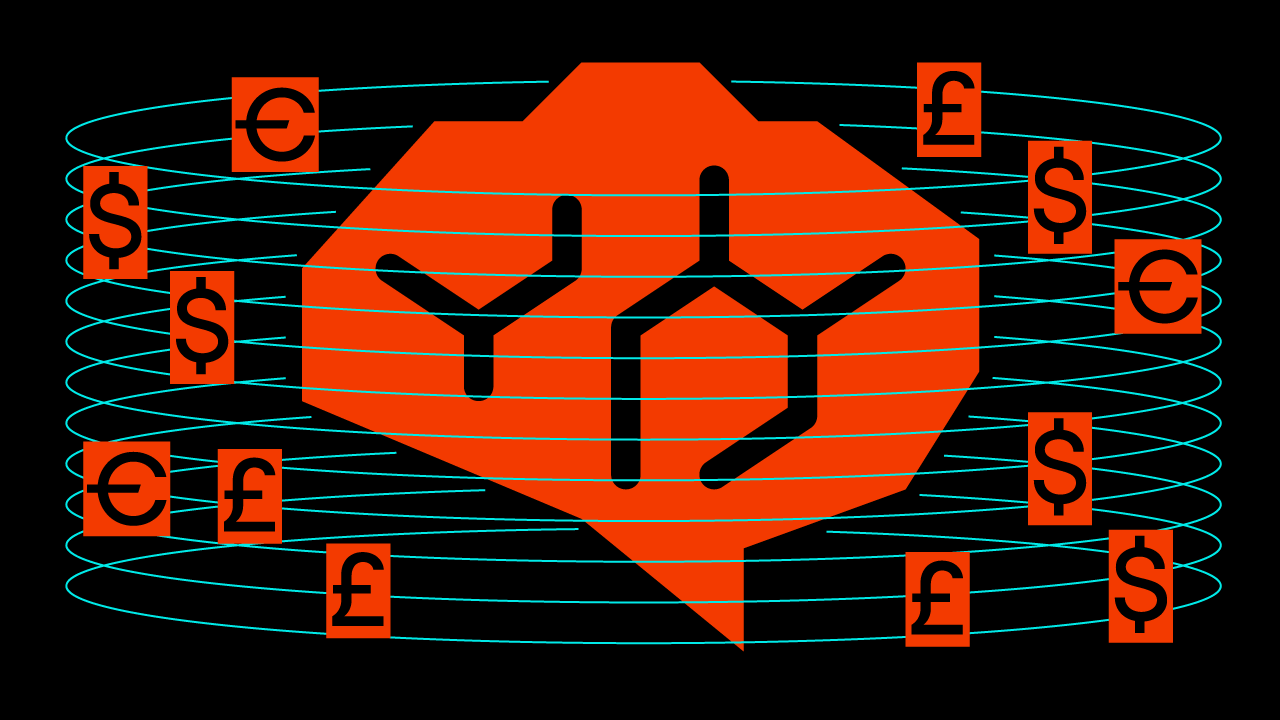
Since the sudden booming popularity of ChatGPT, artificial intelligence tools have become common assistants for personal and professional use. The role of generative AI in banking and other sectors cannot be underestimated – it handles routine tasks, increases employee productivity, helps with content generation, improves customer service, and supports decision-making processes.
In this article, we’ll have a closer look at how AI helps banks curate personalised banking experiences for their consumers. In times of enormous demand for customised interactions with brands, algorithms become allies of financial institutions that want to build loyalty-based long-term relations and keep client retention at the desired level.
Overview of Generative AI Technology
Generative artificial intelligence (or gen AI for short) refers to a class of AI based on large language models designed to create new ideas, content, and solutions by learning from existing data. Unlike traditional AI systems that rely on predefined rules and algorithms, generative AI technologies use advanced techniques like machine learning, neural networks, and deep learning to understand and mimic human-like creativity. These capabilities enable successful gen AI apps to produce text, images, music, and entire digital experiences tailored to specific needs and preferences.
In the banking sector, generative AI has the potential to revolutionise how financial institutions interact with their customers. By leveraging vast amounts of customer data, such algorithms can create highly personalised banking experiences that enhance customer engagement, satisfaction, and loyalty. This transformation is driven by gen AI’s ability to analyse data, generate tailored financial products and services, improve risk management, enhance fraud detection, and more. As a result, AI provides the possibility to embrace entirely new business models and prepares banks for various economic scenarios.
Importance of Personalisation in the Banking Industry
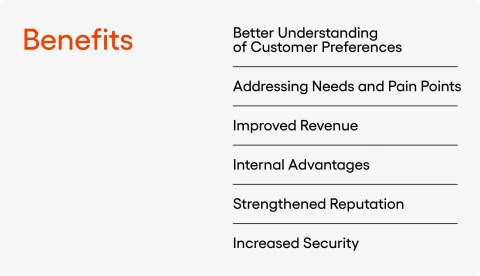
One of the reasons why the financial services industry should invest in personalisation efforts is the increasing demand for personalised business models, as customers seek more relevant financial solutions. In today’s competitive banking industry, customers expect their financial advisors to understand their unique requirements and provide accurate products and services that can solve their problems. Personalisation in banking involves understanding each customer’s financial goals, spending habits, and risk tolerance to deliver financial advice, product recommendations, and marketing messages crafted specifically for them.
Personalised banking experiences offer numerous benefits to both customers and financial institutions:
- Better Understanding of Customer Preferences: Having insight into individual preferences and financial history enables banking companies to build stronger relationships with their customers.
- Addressing Needs and Pain Points: Customers gain services and products they truly need, adjusted to their current challenges or objectives.
- Improved Revenue: Bank employees can preserve profitability and grow conversion rates by responding to the most pressing demands of consumers.
- Internal Advantages: A personalised approach to customers can positively influence operational costs and efficiency, especially when powered by AI.
- Strengthened Reputation: Many banks that focus on personalisation receive better reviews and positive word-of-mouth recommendations.
- Increased Security: With AI-driven models in place, fraud detection and other safety measures are more effective, making risk management more successful.
How Generative AI Enhances Personalisation
Here’s how gen AI algorithms are utilised by banks and financial companies to ensure a high level of personalisation in all communication channels used to interact with customers.
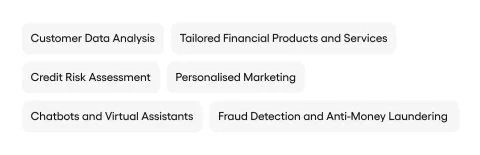
Customer Data Analysis
Generative AI excels in analysing vast amounts of structured data and unstructured data to uncover historical patterns, extract insights, and provide accurate predictions. Data analytics is the most crucial phase of the entire AI-powered personalisation process.
By leveraging machine learning algorithms and deep neural networks, generative AI can process both structured and unstructured data, including transaction history, social media activity, and demographic information. This comprehensive analysis allows banks to gain a deeper understanding of their customers, resulting in more personalised experiences.
Tailored Financial Products and Services
With the help of gen AI, banks can create custom-made financial products and services that meet the unique needs of each customer. For instance, an AI-driven model can analyse a customer’s spending patterns and risk tolerance to provide personalised loan underwriting, recommend credit card options, and offer investment strategies. This level of customisation ensures that customers receive relevant and valuable financial solutions that align with their goals and capabilities.
For instance, AI-driven wealth management apps illustrate how such tailored services work – bots can support customers in not only monitoring their accounts but also in achieving their investment and savings goals. Each user gets individual treatment and personalised advice.
Credit Risk Assessment
Generative AI can enhance credit risk assessment by analysing a wide range of data points, including transaction history, credit scores, and money transfer patterns. Thanks to advanced machine learning models, AI can provide more accurate and comprehensive credit risk exposure assessments than human representatives, enabling banks to make better-informed lending decisions faster. Banks can identify potential risks more accurately and efficiently, while also reducing default rates and proposing offers for customers previously overlooked by traditional credit assessment methods.
For example, loan applicants with a strong credit history and a high-risk tolerance might receive recommendations for better offers, while customers with a lower risk tolerance might be advised to consider more conservative options. This tailored approach not only enhances customer satisfaction but also improves the likelihood of customers taking up the recommended financial products.
Personalised Marketing
Preparing customised content, email campaigns, app notifications, and marketing messages has revolutionised sales and marketing business units in the banking sector. By analysing historical data patterns, a gen AI-driven model can create highly targeted and relevant marketing content that resonates with individual customers.
This level of personalisation can significantly improve the effectiveness of marketing campaigns, leading to higher engagement and conversion rates. Additionally, AI can distribute marketing materials across sources, supporting omnichannel strategies without compromising the individual treatment of new or potential customers.
Chatbots and Virtual Assistants
Generative AI-based chatbots, voice bots, and virtual assistants can provide personalised customer support 24/7, offering instant responses to customer inquiries and resolving issues in real-time. These AI-driven solutions can understand and respond to customer queries in a human-like manner, providing personalised recommendations, financial advice, and support. This not only enhances the customer experience but also reduces the workload on human customer service representatives, improving operational efficiency.
For instance, a generative AI-powered bot can assist customers with tasks such as current account balances, solving technical difficulties, or learning about the bank’s offers. With the right prompt engineering and suggestions, such bots can be highly accurate and precise. The examples of such solutions include Cora from NatWest and Bank of America’s Erica.
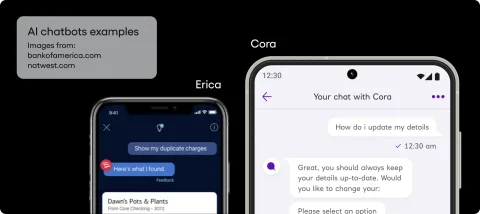
Fraud Detection and Anti-Money Laundering
Fraud detection and prevention become much more effective when a gen AI-driven model analyses user behaviour and transaction patterns in real-time. Advanced machine learning algorithms identify unusual patterns and anomalies that may indicate fraudulent activity – they can even detect synthetic fraudulent transactions. A proactive approach based on gen AI allows banks to minimise and eliminate fraud more effectively, protecting both the institution and its customers from financial losses.
Usually, the AI-driven system monitors transactions and flags suspicious activities, such as sudden large withdrawals or atypical purchase locations. Banks can take immediate action to protect their customers’ accounts, and personalised safety measures are more successful thanks to quick reactions enabled by AI.
Besides fraud detection, a generative AI-driven model can also play a crucial role in anti-money laundering efforts by analysing large volumes of transaction data to identify potential illegal activities. Algorithms can detect and flag patterns indicating such transactions, enabling banks to comply with regulatory requirements.
Examples of money laundering include multiple small transactions below reporting thresholds or transactions involving high-risk countries. By detecting these suspicious transactions, artificial intelligence can help banks meet legal obligations necessary to run financial operations.
Implementing Generative AI in Banking
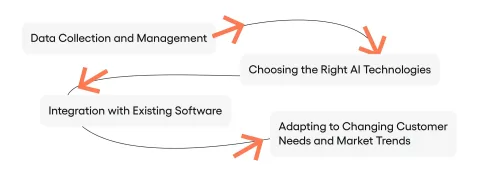
To achieve the highest level of personalisation and introduce all AI-driven features into a banking solution, several steps must be taken. Here’s the process of implementation explained step by step:
Data Collection and Management
To effectively implement gen AI, banks must collect accurate data, prepare proper data architecture, and ensure its management meets data privacy measures and regulatory compliance. This includes establishing robust data governance frameworks, implementing data encryption and anonymisation techniques, and obtaining customer consent for data collection and usage. By prioritising data security, banks can build trust with their customers and ensure they meet the requirements of laws like GDPR and CCPA.
Data collection and management are critical for the success of generative AI in banking sector. Accurate and comprehensive data allows an AI-driven model to learn better and then create relevant insights, while data privacy measures ensure that customer data is protected and used ethically.
Choosing the Right AI Technologies
Selecting the appropriate AI tech stack is crucial for successfully implementing generative AI in banking. Financial services companies should collaborate with seasoned outsourcing agencies specialising in AI development to identify the most suitable tools and frameworks for their specific needs. This may involve utilising a pre-trained gen AI-driven model, developing custom solutions, or integrating AI capabilities into existing systems. For example, Danske Bank is in the process of preparing several internal AI products, and they use proven technologies like GPT, Microsoft 365 Copilot, and GitHub Copilot.
By choosing the suitable AI technologies, banks can ensure that their generative AI solutions are effective and scalable. This involves evaluating various AI platforms, assessing their capabilities, and selecting the ones that best meet the company’s requirements. Having a trusted AI partner is the best strategy to eliminate potential difficulties in that area.
Integration with Existing Software
Integrating gen AI technology with existing banking processes seamlessly is essential to fully embrace innovation. AI capabilities can be added to customer relationship management (CRM) systems, core banking platforms, and digital channels. By ensuring smooth integration, banks can maximise the benefits of generative AI and deliver personalised experiences across all customer touchpoints.
Effective integration of generative AI with existing systems requires careful planning and execution. Banks and their development vendors have to assess the compatibility of generative artificial intelligence with existing systems and develop integration strategies that won’t be too disruptive for ongoing operations.
Adapting to Changing Customer Needs and Market Trends
Customer preferences are continuously changing. To stay competitive, banks must adapt to these changes by continuously refining their generative AI solutions and personalisation strategies. Monitoring market trends, analysing customer feedback, and updating the operating model to ensure it remains relevant and effective is a crucial strategy to follow.
By staying on top of the evolving market situation, banks can ensure that their gen AI tools continue to deliver value and enhance the customer experience. BNP Paribas is an example of a bank that uses AI to listen to their clients and introduce new solutions accordingly.
Challenges of Generative AI in Personalised Banking Experiences
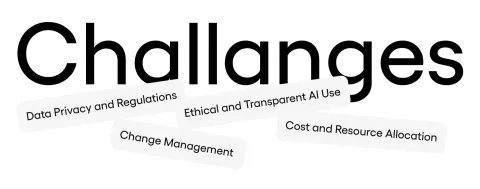
Like any other technological advancement, generative AI-driven personalisation comes with its own considerations. Here’s what to take care of when implementing the above-mentioned approach:
Data Privacy and Regulations
Compliance with data privacy regulations is crucial when using data for AI-driven personalisation. Financial institutions must implement robust data protection measures, obtain customer consent, and maintain transparency in data usage. Banks should also regularly review their data practices to ensure they meet the regulations at all times.
For now, the regulation landscape regarding generative AI in banking is still unclear. It lacks many necessary documents. However, many institutions continue working on the development of AI-related acts to quickly introduce relevant guidelines and rules. Currently, financial organizations prefer to test AI internally instead of using it in external products and services to not risk AI hallucinations in contact with consumers or risk their personal data. Citi is an example of a bank that invests in AI-driven initiatives, but withholds them on front to make sure AI is reliable enough.
Ethical and Transparent AI Use
Addressing biases in an AI-driven model and promoting fairness in results produced by generative algorithms is crucial for maintaining customer trust and avoiding reputational damage. Banks must implement transparent AI practices, conduct regular audits of AI models, and take steps to mitigate biases in AI-generated recommendations and decisions. They can do this by using diverse training data, monitoring AI outputs, and involving human expertise in critical decision-making processes.
Clear communication with customers about implemented generative AI tools is essential for building trust and confidence. Banks should clearly explain how AI is used to personalise experiences, the benefits of an AI-driven approach, and the measures taken to protect customer data. Providing customers with control over their data and the ability to opt-out of AI-driven personalisation can further enhance trust and satisfaction.
Cost and Resource Allocation
Implementing generative AI in banking requires significant investment in technology, data architecture, tech infrastructure, and talent. Banks must allocate sufficient resources to develop, deploy, and maintain gen AI applications while ensuring a positive return on investment. Hiring data scientists and skilled AI development experts, as well as investing in relevant technology architecture, is the best way to address this challenge.
Change Management
Successfully implementing gen AI in banking requires effective change management to address the difficulties associated with technology adoption. Financial organisations must provide training for employees, foster a culture of innovation, and address signs of resistance proactively. By involving employees in the AI implementation process and providing them with the necessary skills and support, banks can ensure a smooth transition and maximise the benefits of AI introduction. Deutsche Bank recognizes how important it is to prepare their crew for AI-driven change and invests their efforts into preparing the right change management strategy.
Influence on Customer Experience
Generative AI has the potential to significantly enhance the customer experience in the banking industry by delivering highly personalised financial solutions. Thanks to advanced AI technologies, banks can achieve the highest level of individualised services ever. This leads to revenue growth for financial institutions and provides a plethora of benefits for their customers:
- Enhanced Engagement: Generative AI can create customised financial plans, investment banking solutions, and risk management advice that capture the customer’s attention and encourage customer interactions.
- Improved Satisfaction: Personalised banking experiences are achieved by providing relevant and valuable financial solutions that meet the customer’s unique needs, increasing overall satisfaction.
- Increased Loyalty: Gen AI models can help banks build stronger relationships with their customers. Individual treatment means that consumers are more likely to recommend a bank that understands and meets their financial needs.
- Better Retention: Clients who feel their banks care for them are more likely to stay with one provider and trust their other services.
- Faster Support: Personalisation achieved with generative AI-driven models allows banks to quickly address requests and solve issues, leading to better reviews and an overall positive overview of customer service.
- Effective Marketing: Companies that deliver personalised messages from the first contact with customers can count on more sales and better ROI.
- Risk Management: Most banks and financial services companies that invest in AI manage risk more responsibly, providing security for their customers.
Personalisation in Banking Case Studies
Gen AI models are poised to revolutionise the banking industry by enabling highly personalised customer experiences. Artificial intelligence was made to analyse vast amounts of customer data and create tailored results – ideas, marketing content, support messages, and proposals adjusted to individual desires. Financial services offered by banks, including loans, investments, and insurance, can benefit from AI implementation. Awareness of challenges means banks have higher chances of achieving success and can embrace the internal advantages, as well as growing satisfaction within the client base.
Many financial companies have successfully utilised generative and distributed AI to boost profits, streamline operations, and improve customer interactions. If you’d like to learn more about the impact of distributed AI on business, visit our detailed page here.